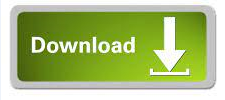

Experiments also showed that Support Vector Machine (SVM) classifier, optimized by Gray Wolf Optimizer (GWO) and using Radial Basis Function (RBF) kernel outperforms the Random Forest classification algorithm with a set of selected features.
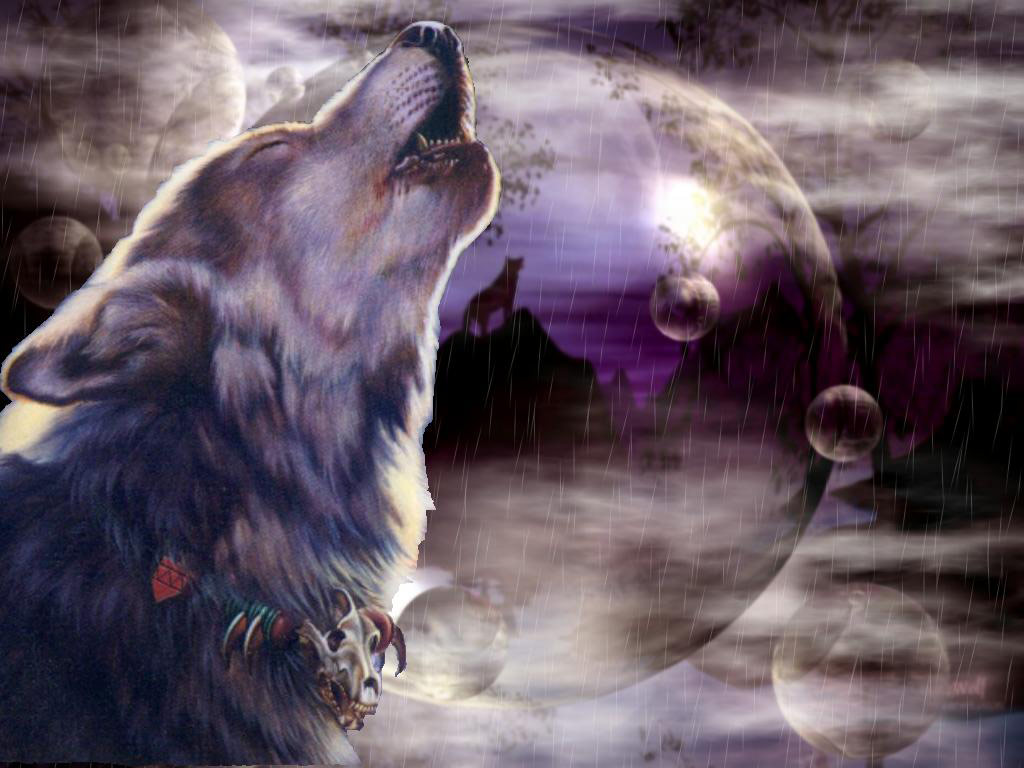
(3) Results: The proposed system based on the improved LevelSet algorithm proved its efficiency in bladder wall segmentation. After an automatic selection of the sub-vector containing most discriminant features, two supervised learning algorithms were tested using a bio-inspired optimization algorithm. Several features were computed from the extracted wall on T2 MRI images. (2) Methods: For each image of our data set, the region of interest corresponding to the bladder wall was extracted using LevelSet contour-based segmentation. This paper proposes an optimized system for the segmentation and the classification of the bladder wall. (1) Background: Segmentation of the bladder inner’s wall and outer boundaries on Magnetic Resonance Images (MRI) is a crucial step for the diagnosis and the characterization of the bladder state and function.
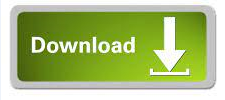